Data has been an important resource in the global fight against the COVID-19 pandemic. Daily tallies of the number of cases and deaths have been integral for predicting the outbreak trajectories location by location. And now, data measuring contact through surveys and digital data from phones are being fed into models to understand the efficacy of social distancing interventions over time in places such as Europe, North America, and China. Diverse places around the world are looking to the results of such models to understand how they may guide policy in prevention and response of COVID-19 in their local context.
In African countries, social distancing was implemented in an average of five days after the first cases was reported
Last month, we started looking at the feasibility of building models to assess the impact of different interventions implemented across the African continent. We aggregated information from multiple websites to assess dates and types of COVID-19 interventions during early stages of the pandemic. We found that policies were generally swiftly enacted. Social distancing interventions, for instance, were implemented within an average of five days after the first cases was reported in African countries—in comparison with European countries, which had an average of thirteen days. Even though the average was good, there was still considerable variation of the timing with which interventions were implemented in relation to cases, which is visible in the map below.
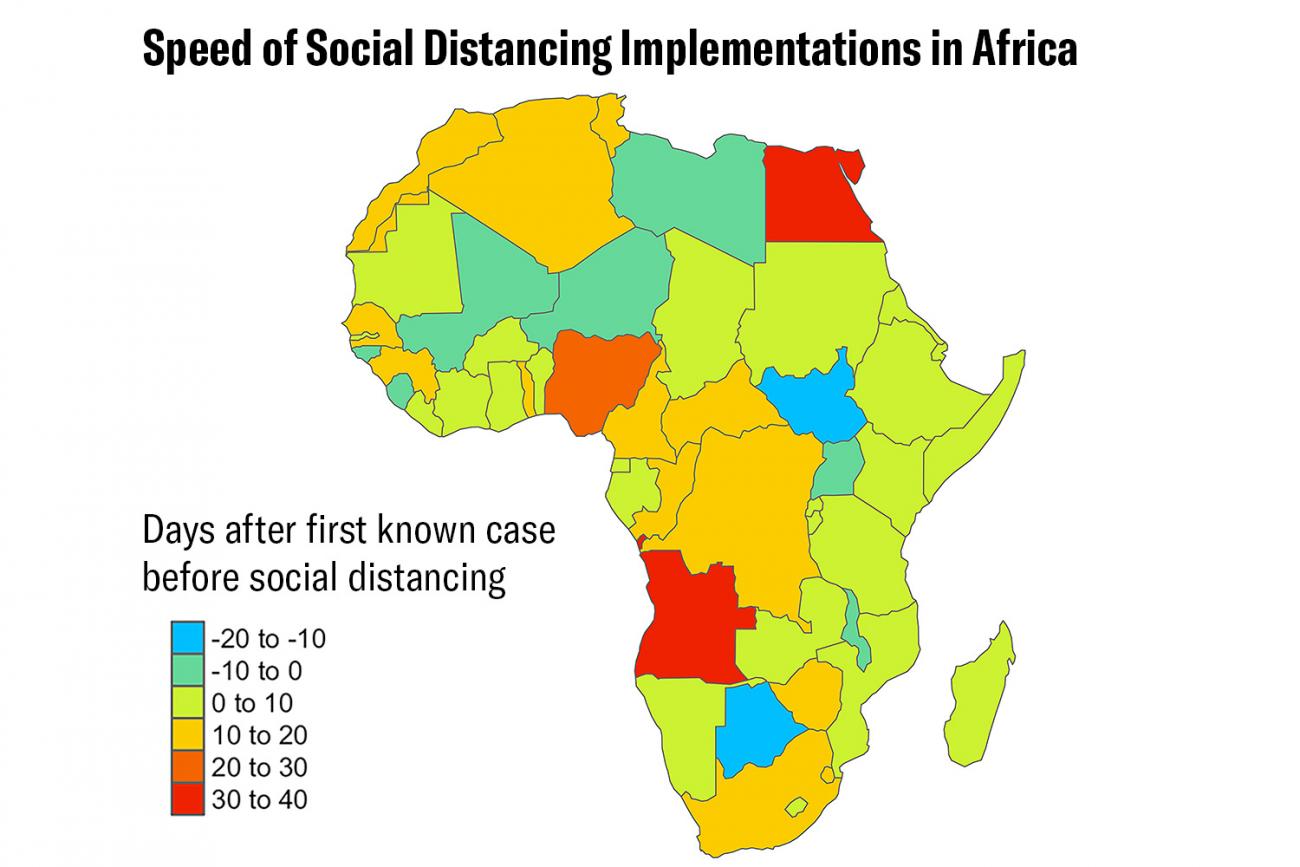
Angola and Egypt had relatively long lags between first reported case and first implemented social distancing intervention, despite having the earliest reported cases in the continent in February—the first reported cases across the continent ranged from February 14 to April 2 (as of writing, Comoros and Lesotho are yet to report any cases).
Policies that result in economic burdens are most difficult to enact by those with the fewest resources
The Democratic Republic of Congo also had a relatively long lag between the first reported case and its social distancing interventions—a night curfew and lockdown were implemented seventeen days after the first case was reported. The last country to enact any interventions was the Central African Republic. Public gatherings and flights were limited nineteen days after the first case. However, due to the country’s heavy reliance on external aid, they could not close flights altogether. This limitation, in one of the poorest countries in Africa, echoes known ethical considerations in mitigating pandemic disease: Policies that result in economic burdens are most difficult to enact by those with the fewest resources.
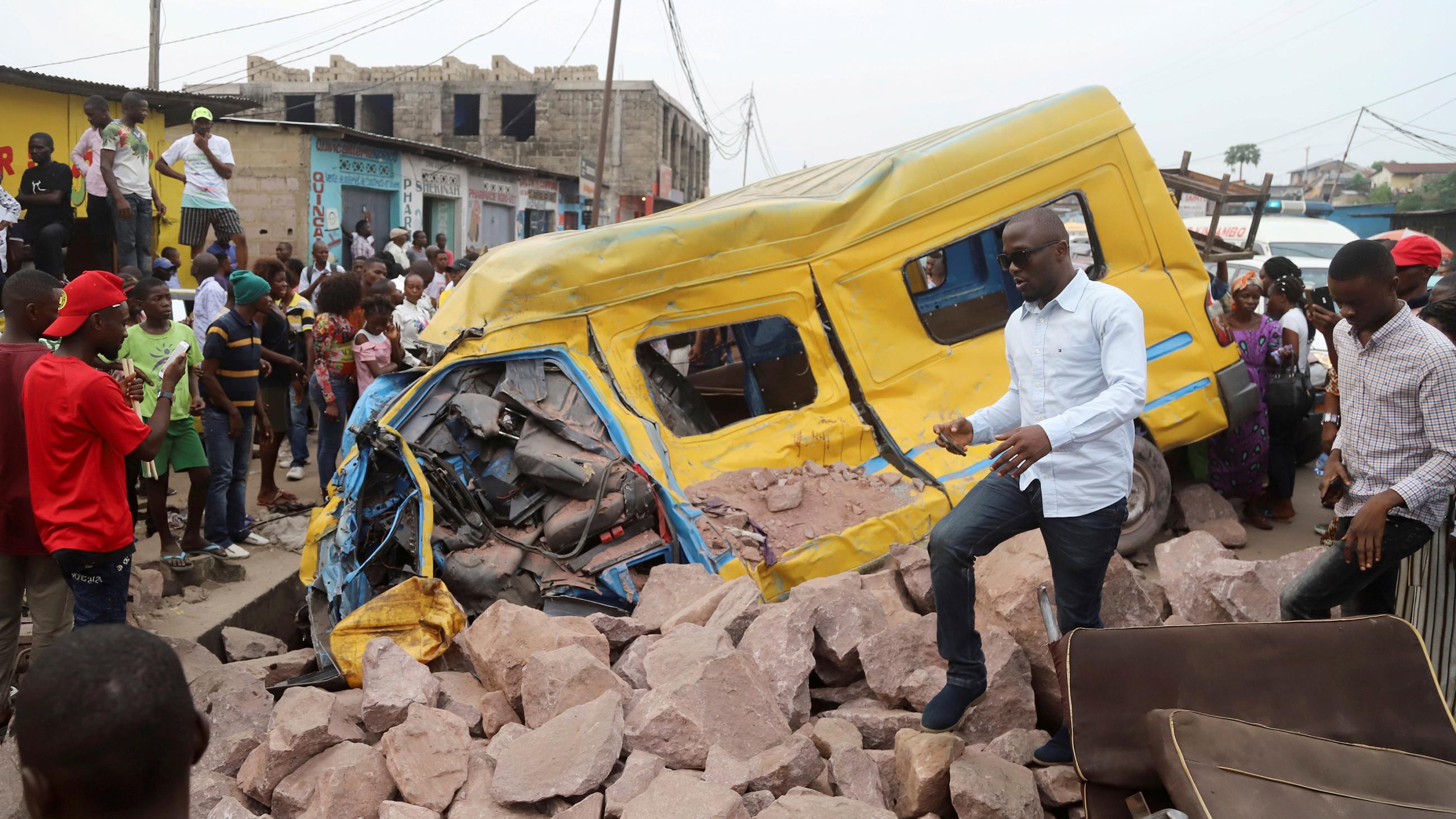
News reports conveyed further tradeoffs and difficulties in implementing interventions even after they were officially instituted. For example, while Lesotho closed schools, they did keep public schools open to continue offering meals for some students who get their only meal at school.
Models built to understand and assess efficacy interventions must also account for their implementation
Other reports indicate how in certain locations, places of worship were disregarding the ban on gatherings. This raises the important point of implementation, and how underlying structural and contextual issues that influence the types of interventions and their timing are also reflected in how these interventions are implemented and maintained. Accordingly, any models built to accurately understand and assess the efficacy of local or state-wide interventions must also account for these factors. Identifying the implementation issues and keeping in mind what kinds of data will be useful in certain contexts can ensure that suitable data is collected and used in a proactive manner.
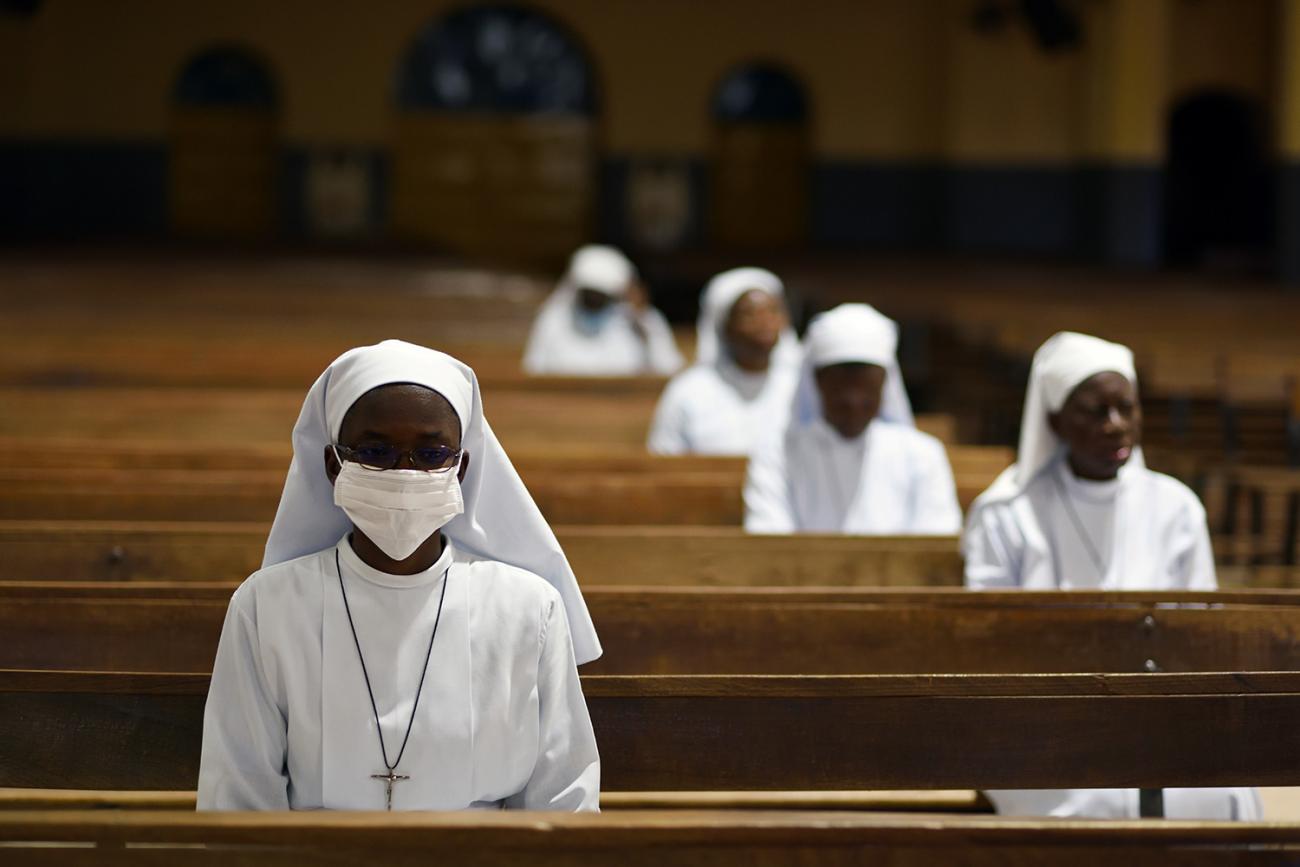
What types of data are needed? We consider three types that, given low-resource situations, will be feasible to collect, will help better understand the dynamics of COVID-19, and define essential parameters in models.
In many countries throughout the world, positive test rates are often a function of test availability and test criteria
First, collecting confirmed data on positive cases is a struggle in COVID-19 efforts even in high-resource countries. In many countries throughout the world, positive test rates are often a function of test availability and test criteria. This is not likely to be much different in African countries. Given that limitations on test availability will likely be amplified, estimates of surveillance capacity should account for what proportion of cases are detected in models. In other words, we should not rely on an actual enumeration of confirmed cases but an evidence-based estimation. Indeed, state-of-the-art modeling efforts assume all cases can be detected or are informed by a specific detection capacity, and an empirical understanding of this variation would improve model accuracy. Just as previous studies have sought to understand health facility access in different parts of Africa, COVID-19 testing availability should also be cataloged via understanding which facilities offer tests and if they are conducting tests and submitting results based on the same criteria. This data would directly quantify surveillance capacity, as well as inform the feasibility of aggregating COVID-19 surveillance data.
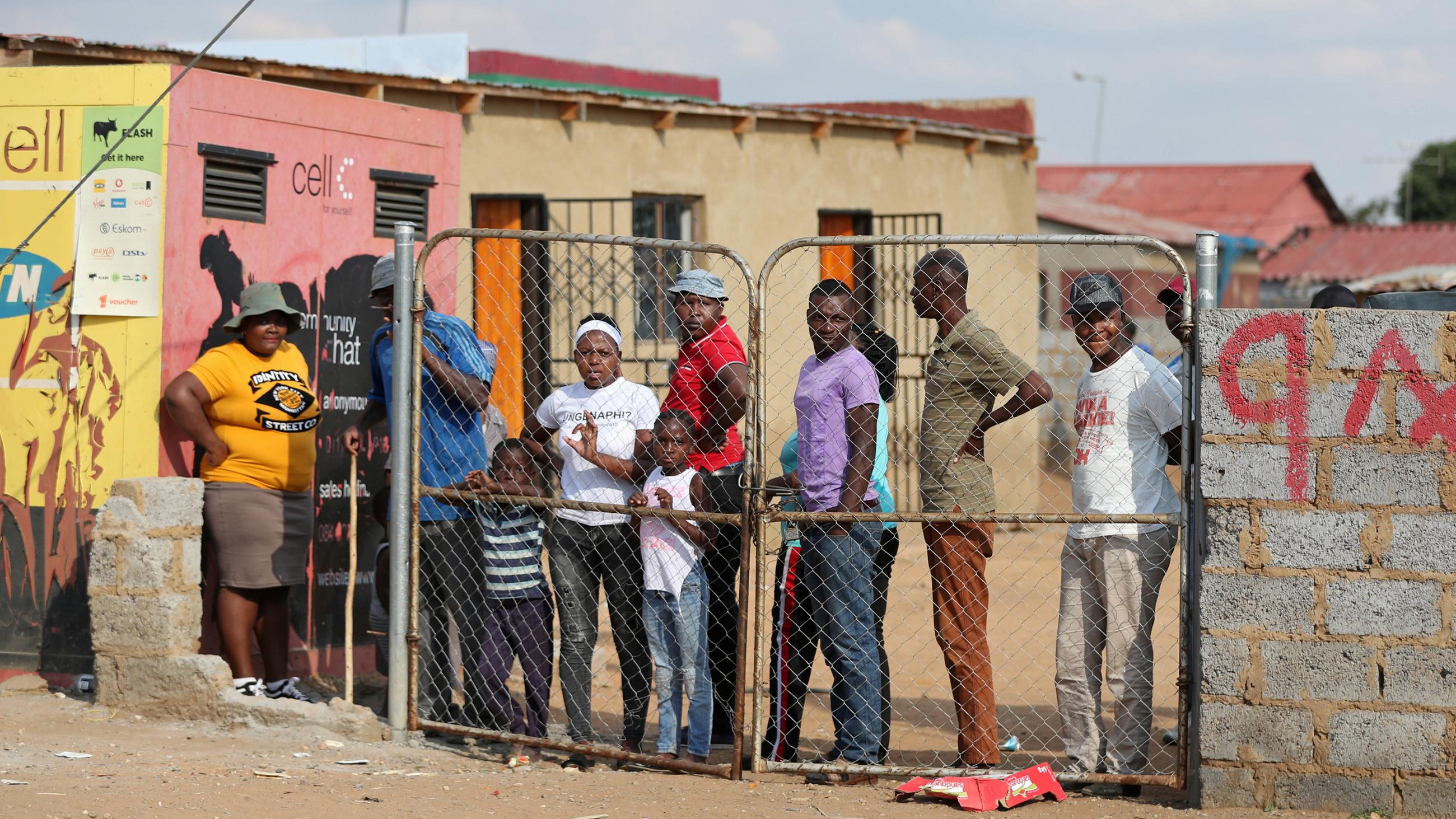
Next, modeling efforts to evaluate the efficacy of interventions have to be informed by information on country-specific factors such as how long it takes those interventions to be put into effect and how well the efforts are maintained. In COVID-19, the use of new data sources such as aggregate GPS tracking from mobile phones is being used to measure and inform evaluations of the efficacy of such interventions. However, as we’ve described above, implementation of interventions, including how well they are maintained, is affected by social, cultural, and economic factors.
These challenges will manifest at the national level in the poorest countries and as well within nations in their poorest parts
In particular, economic instability in places across Africa will severely limit how well individuals and communities are able to adhere to such interventions. These challenges will manifest at the national level in the poorest countries and as well within nations in their poorest parts. Additionally, leveraging data that requires technology access, such as using smartphones for geo-location tracking will be challenging for the most vulnerable locations. These disparities are exemplified in Google’s mobility data, released to aid in assessment of social distancing interventions, which is only available for twenty-six countries in Africa, and only at the subnational level for four countries. Notably, some of the poorest places (countries such as the Democratic Republic of Congo), or poor places within countries will be omitted. Thus, other ways to get data to catalog the impact of social distancing interventions must be enacted locally, such as through targeted surveys, or including this as part of community health worker assessment practices. Even capturing such information from a sample of regions can be used to inform targeted public health messaging, optimizing resources.
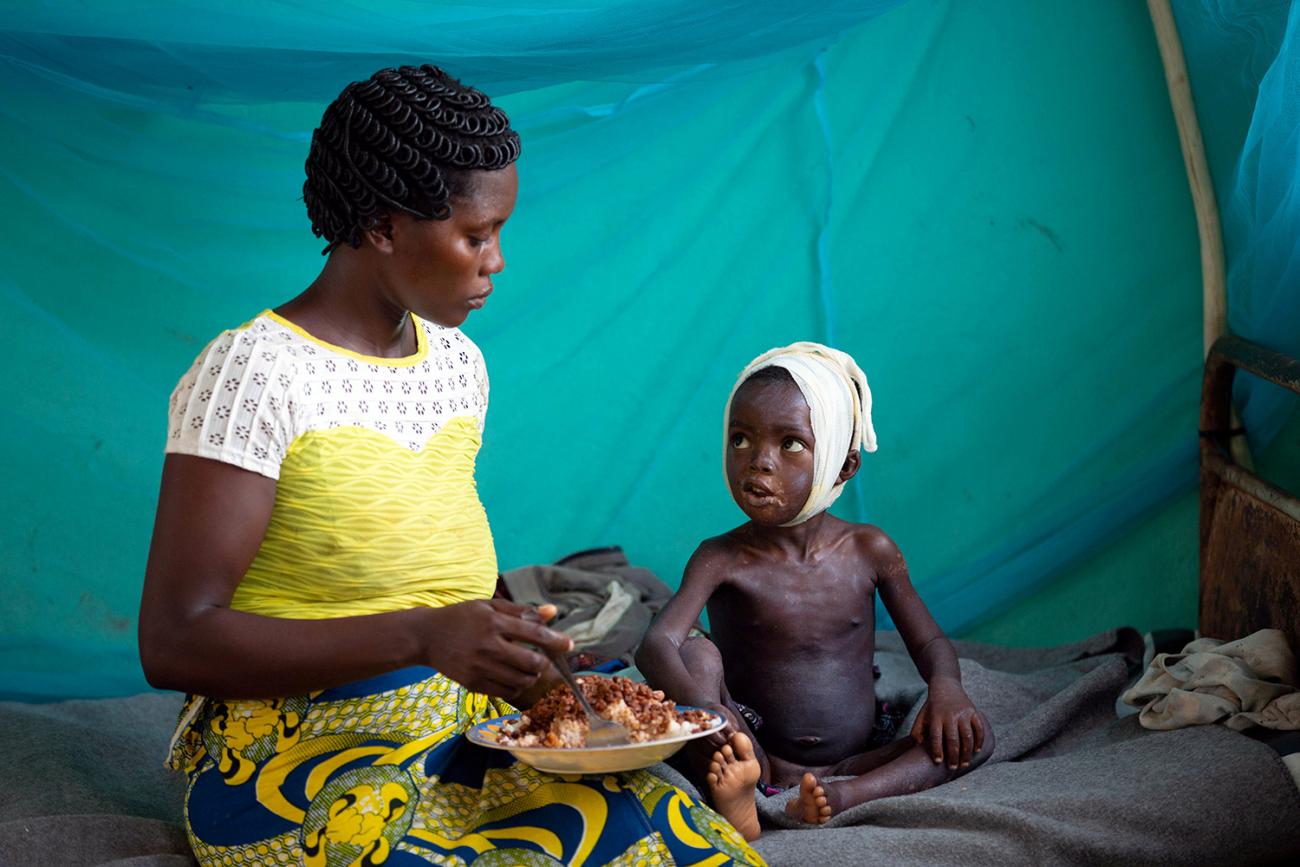
Finally, current conditions of economic deprivation, sociocultural challenges, and the burden of other diseases in Africa means that many places have extreme vulnerabilities, which COVID-19 incidence and interventions such as social distancing may exacerbate. For instance, there is already worry that millions of children will miss out on measles vaccines during COVID-19. In order to proactively mitigate effects of refocusing the already limited healthcare resources on this pandemic away from other critical and contemporary economic and health burdens, data can be collected to understand and plan such shifts so that overall burden is minimized. This would mean, for instance, recording the reallocation of resources for surveillance and health care capacity from other disease programs to COVID-19.
Surely, the issues around data quality described here may not all be specific to Africa
Data on interventions enacted to date show it is clear that the potential impact—directly from COVID-19, and due to disparate implementation and maintenance of restrictive public health measures—may have disproportionately devastating impacts on vulnerable populations. Data can be a powerful resource, and the types of data collected can be adapted by context in order to inform and prioritize models that are most important to the context. Surely, the issues around data quality described here may not all be specific to Africa—in general, instead of using the same data in the same ways it is used in other places, awareness of local challenges can help us collect data strategically to illuminate and address challenges. This can help work toward leveraging data as an asset to be trusted and used for COVID-19 and beyond.