As artificial intelligence (AI) comes into broader public awareness, questions have mounted regarding its ethical and effective use. Global survey data on trust and adoption indicates growing consumer curiosity, but also expressions of fear and nervousness resounding in public discourse.
Although concerns regarding risks and harms are viable and should not be understated, generative AI also offers an optimistic counterpoint: underexplored opportunities to conceptualize better, brighter futures. By motivating people in unprecedented ways, generative AI could help mobilize progress in addressing the most persistent, pervasive challenges of our time: compounding threats to global health and environmental security.
These threats are especially salient in light of the COVID-19 pandemic, which magnified critical fault lines and exposed the need for improved health system resiliency across country contexts. Among other pushes for health system transformation, significant investments have been made to explore how AI can enhance everything from direct patient care to system-level processes. Routinely used throughout the health-care ecosystem, AI-enabled tools already support data management and integration, streamline care coordination, and predict patient needs to inform clinical decisions.
Beyond these and other applications, generative AI could further push the field by fueling creativity, imagination, and innovation in how we respond to exigent public and environmental health crises.
What Is Generative AI?
Broadly speaking, generative AI is functionally using machine-learning models to develop new content rather than solely producing predictions about the inputs on which a system was trained. Among other things, generative AI enables and expedites real-time and responsive creation, revision, and synthesis of information.
It facilitates tasks that would otherwise be time intensive and technically or artistically challenging, which affords reimagining or re-envisioning at an almost fantastical scale: sourcing and synthesizing unfathomable amounts of data, designing art and architecture that defies laws of physics, creating whimsical worlds and creatures that seem scientifically nonviable—at least for now.
Climate Action and Health Promotion: The Problem of Present Bias
Where existing knowledge, methods, and imaginations bind us, generative AI could enhance our ability to grasp the scale of change, expanse of space, or passage of time. It could thus be a powerful catalyst in the fields of public health and health promotion, which have long grappled with how to address the lack of personal accountability for public and environmental health threats.
Where existing knowledge, methods, and imaginations bind us, generative AI could enhance our ability to grasp the scale of change
Because gradual public and climate health shifts can be hard to perceive or detect through personal experience, we tend to underestimate how emergent threats affect our present or future lives. We also tend to distance or dissociate from these issues when struggling to feel hopeful or optimistic that our individual actions can help address threats of global magnitude.
To successfully overcome ambivalence or disengagement, health promotion and climate action campaigns should not only appeal to fact or logic but also account for psychosocial drivers of decision-making. In these realms, beliefs about self-efficacy (the confidence in one's ability to adopt suggested behaviors) or outcome efficacy (the confidence that one's adoption will have desired consequences) moderate the effectiveness of calls to action. Absent consideration of these factors, behavior change campaigns falter as people report feeling disconnected from intangible future selves or ecosystems and overwhelmed by global or diffuse challenges that seem indirectly linked to their choices.
This perceived disaggregation between local actions and distant or abstract consequences has undermined calls, for example, to observe pandemic-era masking practices or reduce carbon emissions. It's partly an artifact of humans' present bias: our natural predisposition to prioritize attention and resources (such as heightened brain activity) to our physical present selves, relative to future selves whose health will manifest in a distant time.
Research suggests, however, that showing people visual representations of themselves later in life can induce near-term behavior change that supports long-term health and well-being. In essence, seeing the future self mobilizes people to empathize with and better care for it. This reinforces suggestions, long echoed in canons of communications and behavior change literature, that images evoke affective reactions and can override emotional disinvestment to prompt otherwise challenging action or reflection.
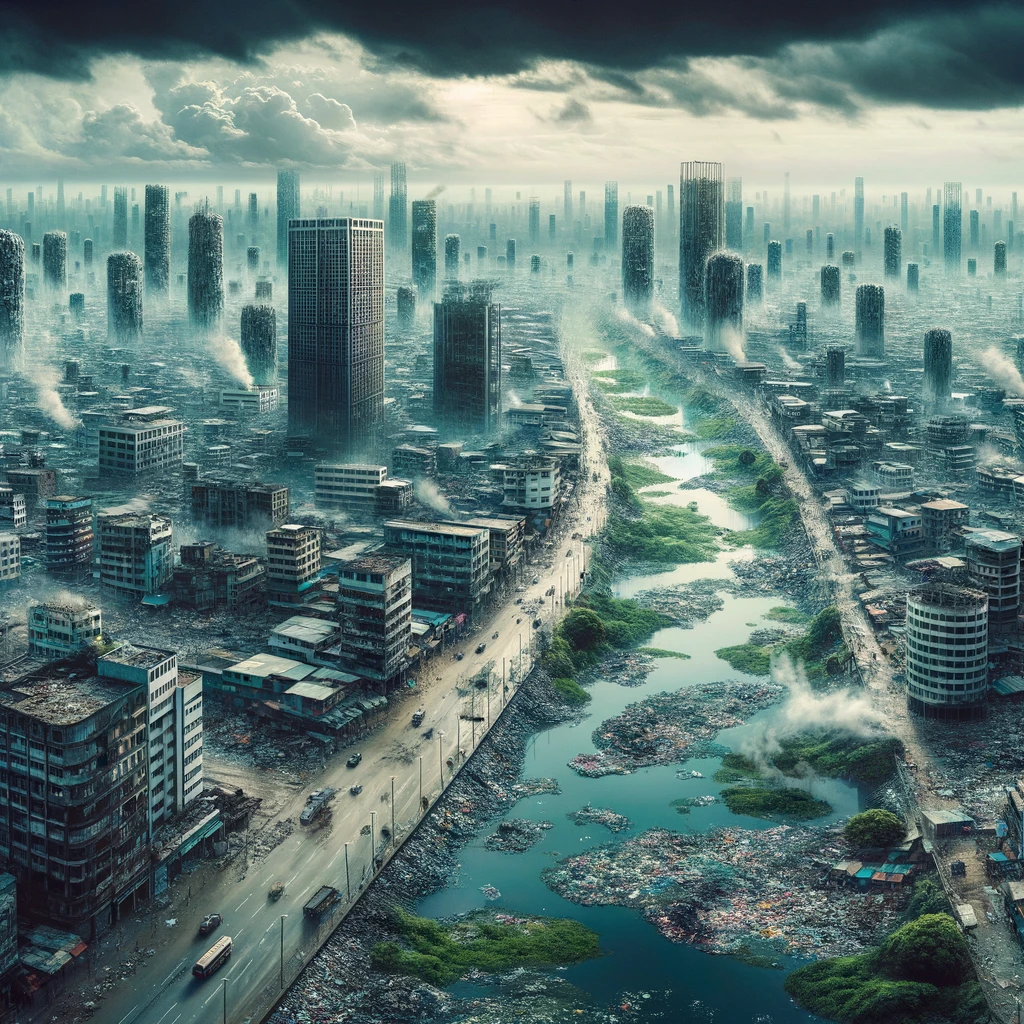
How Can Generative AI Help?
If AI-generated images help people consider the health of their future bodies, can they also prompt thinking about their future communities or environments? Or excite people about what those might look like, if properly nurtured and preserved? In a critical moment when immediate action should be taken to promote health and environmental system resiliency, generative AI might increase personal urgency and agency to adopt health- and environment-preserving actions in at least two ways.
Anticipating harms. As of August 2023, more than 15 billion visuals had been generated using text-to-image models such as DALLE-2, Midjourney, Stable Diffusion, and Adobe Firefly. In the realms of both medical and environmental research, synthetic images already inform intervention planning, enable advanced scientific simulations, and aid harm detection or disease diagnosis. For example, UK-based Utility Bidder recently released Midjourney-generated images foreshadowing how well-known global sites may deteriorate unless people adopt environmentally sustainable habits.
Notably, these images are harrowing and resonant but not altogether shocking. Evidence suggests the need for caution because overly negative visuals can have inverse effects, rendering people defensive or inert. Referencing a public health example, shock-fueled smoking cessation campaigns were deemed broadly successful in decreasing overall smoking rates. Evidence indicated, however, that overtly threatening or disgusting images had a boomerang protective effect.
Eliciting intense visceral reactions such as fear or overwhelm often stopped people's mental processing, leading to desensitization and the loss of core messages. By evoking a sense of fatalism or learned helplessness, vivid images that are not carefully curated could yield deeper dissociation or further inaction.
Imagining possibilities. Rather than rely on retroactive images of a diseased lung or foreboding images of a charred landscape, AI-generated artwork could spark hopefulness and optimism about future global health and broader ecosystems. Imaginative or even beautiful content might expand people's thinking about what's possible for healthy future lives and landscapes, increasing personal ownership or investment in that shared future.
Although potentially powerful, these use cases warrant crucial caveats
Creating these images is one of innumerable generative AI applications for the field, from suggesting uncharted research topics or hypotheses, to facilitating citizen engagement, and even to proposing new models and frameworks for understanding the world. AI-driven technology may even hold promise for mitigating inequities. Although lower-income countries in the Global South experience disproportionately negative health outcomes (and brace for growing disparities in light of climate change), they also have the highest rates of reported optimism regarding AI's potential positive impacts. Where resource or geopolitical constraints could limit ideation or innovation, AI-driven platforms could help cultivate it.
Considerations for Responsible Use
Although potentially powerful, these use cases warrant crucial caveats. International regulation of AI-generated content is plagued by challenges not dissimilar from those experienced in the spheres of environmental or global public health. Digital content has global reach, drivers of change span country borders, and the rapid development and diffusion of new technology begets a volatile landscape. Despite intergovernmental movement toward establishing AI governance models, it remains difficult to ascribe credit (or assign liability) for content. Thus it is crucial that content creators and disseminators take several personal responsibilities seriously.
Present synthetic images as balancing accuracy and artistic license, at the discretion of the prompt engineer. Generative adversarial networks commonly used for AI-enabled image creation rely on generator and discriminator neural networks working in tandem. The generator, having been trained on real data, learns to create new and seemingly realistic or plausible data points. Then, by "learning" to distinguish between real data points and these artificial alternatives, the discriminator is trained to rule out utterly implausible data. In the case of image generation, the result is production of visuals that are imaginative and innovative but still subject to realistic boundaries. This dynamic prevents contrived images from becoming altogether otherworldly and makes them especially difficult to discern from actual photographs.
Provide transparent information about images' intended use. To avoid misleading viewers and contributing to the spread of misinformation or misunderstanding, creators should disclose whether content was designed as purely creative or as potentially predictive. Is this an AI-supported restoration of a real video or a fully manufactured scene? To what extent is this image an accurate indicator of some future state? Or is it intended primarily as a piece of art, designed to prompt imaginative thinking about what could be?
Communicate clearly about inherent biases. Responsible creation and use of this content requires explicitly reinforcing that AI-generated or -enhanced artifacts naturally perpetuate bias of the content creators or underlying algorithms, and embedded in the bodies of art, evidence, or information on which those algorithms are trained. Further, because this work is bound by current ideas about unknown or utopic futures, realities projected through these images are subject to evolve—just as the world will evolve beyond how we currently experience it.
Although that evolution is inevitable, adopting these and other best practices can increase AI literacy and equip people to interpret and interact with content in informed ways. Promoting trust and alleviating fears will invite viewers to join the work of ideating about a vision for a healthier shared future that they are eager to personally protect and preserve.
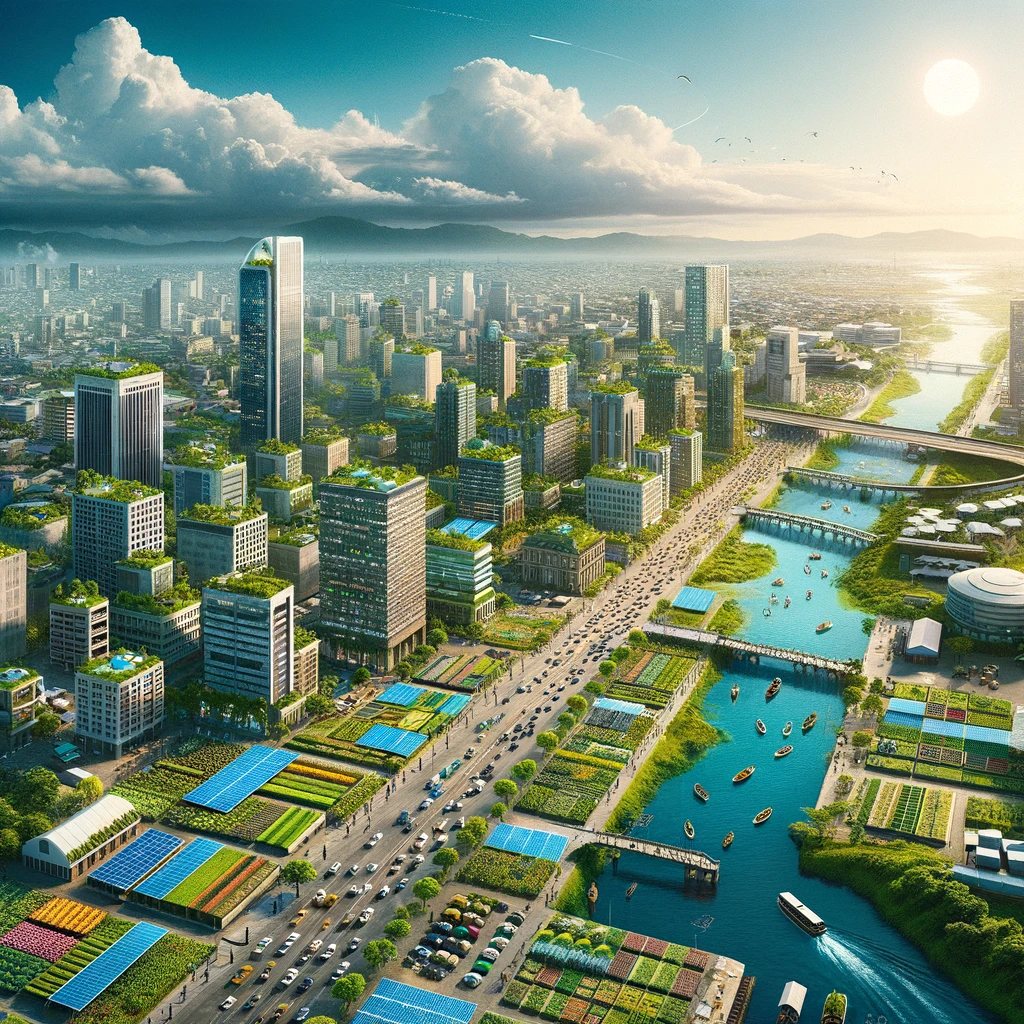
EDITOR'S NOTE: This article is part of a series exploring the ways artificial intelligence is used to improve health outcomes across the globe. The rest of the series can be found here.