The ongoing COVID-19 pandemic has revealed that many countries have limited capacity to reliably collect high-quality data and conduct analysis to detect, monitor, and report on diseases with epidemic potential. All faced with a novel disease, COVID-19, health systems around the world have had to scramble to answer very basic questions: how many people are infected, how many have recovered, and how many have died from the disease? Underlining the challenge to low- and middle-income countries, where resources are scarce, is the fact that gathering useful data has been a challenge in many high-income countries as well.
Data should flow freely, like water, across health systems, allowing analysis, reporting, and evidence-based decision-making
Disease surveillance and effective responses depend on having data to guide decision-making at various levels of the health system—high-quality data, which should be accurate, complete, timely, valid, and reliable. In addition to these quality criteria, the data should be open. Data should flow freely, like water, across health systems, allowing researchers to analyze it, the media to report it, and decision-makers to use it as evidence to guide policy. Transparency in health data systems allows for faster detection of epidemics and decisive monitoring of the impact of control measures, such that appropriate corrective actions are implemented as needed. The Ebola epidemic of West Africa that ravaged the region between 2013 and 2016, is a constant reminder that weak health data systems often translate to delayed action, leading to negative health and socioeconomic consequences. The rapid spread of the Ebola outbreak from Guinea, where it started, to Liberia and Sierra Leone where it had devastating consequences, was largely attributed to the fact that the health systems in place in the three countries lacked the infrastructure and the preparation to detect the epidemic and respond. Now, with the COVID-19 pandemic ravaging countries around the world, there is a sense of déjà vu that global health systems did not adequately internalize the lessons of the Ebola epidemic.
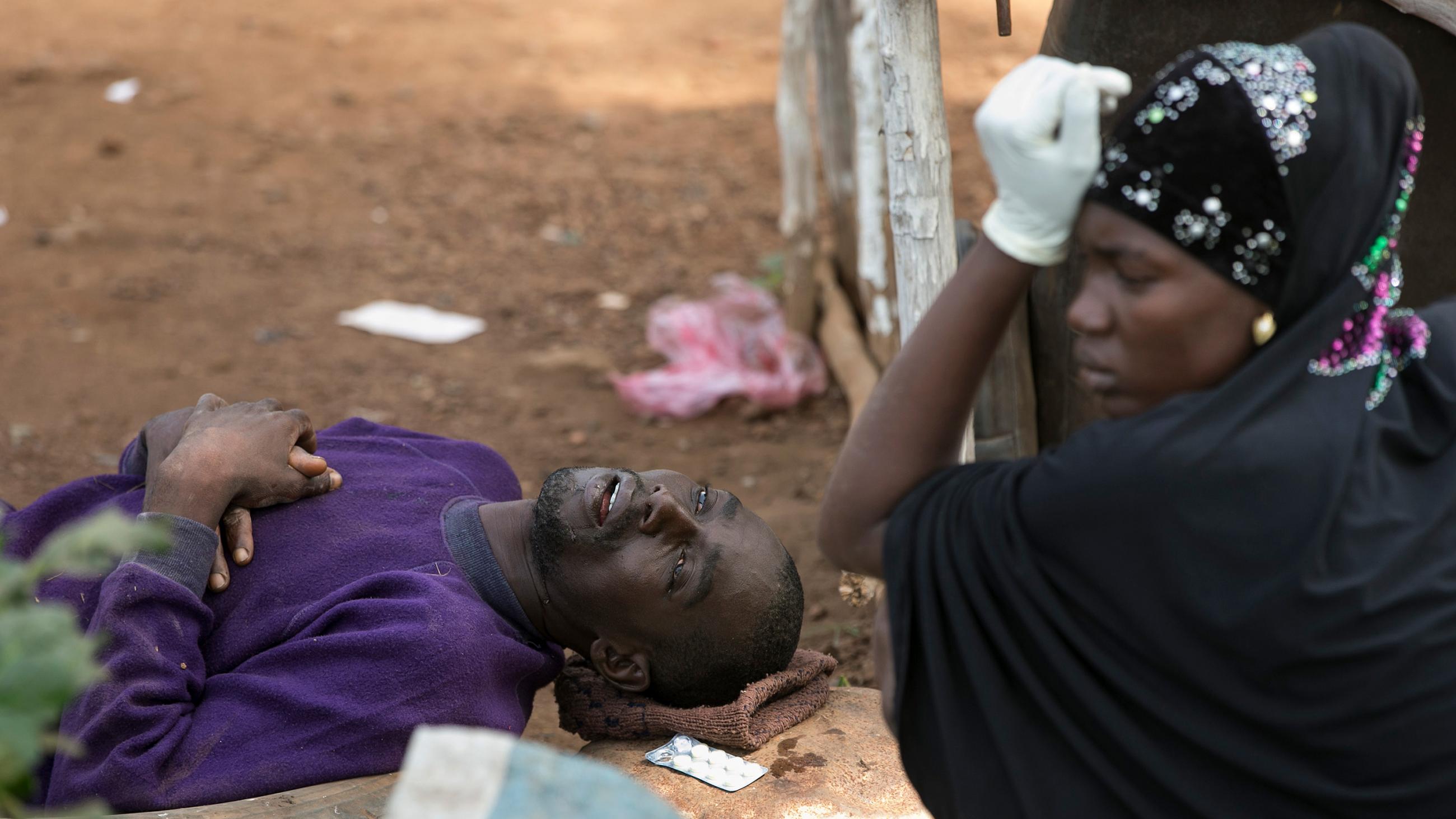
There are many interrelated factors that contribute to the observed weaknesses of health data systems: weak data governance systems and organizational challenges, poor system design, structural and implementation pitfalls, human and financial resource constraints, and inadequate or inappropriate technological adoption—among others. These ultimately impose multiple bottlenecks in the flow from health data to decision-making, effectively hampering critical steps in data gathering and its translation into actionable evidence. To fully understand the underlying processes, it is imperative to appreciate the stakeholders involved in the health data landscape.
These ultimately impose multiple bottlenecks in the flow from health data to decision-making
In their own health journeys, individuals often interact with various parties including clinics, hospitals, pharmacies, diagnostic laboratories, insurance companies, as well as community health systems. Beyond the health care system, people also engage with organizations such as housing authorities, retailers, and transportation systems that have a direct or indirect impact on health. These interactions usually produce copious amounts of data that can be useful in health care decision-making. The value of this data is even more amplified when analyzed collectively rather than in isolation, because it can illuminate causal linkages, revealing behaviors, and hidden associations that guide health care decisions at various levels.
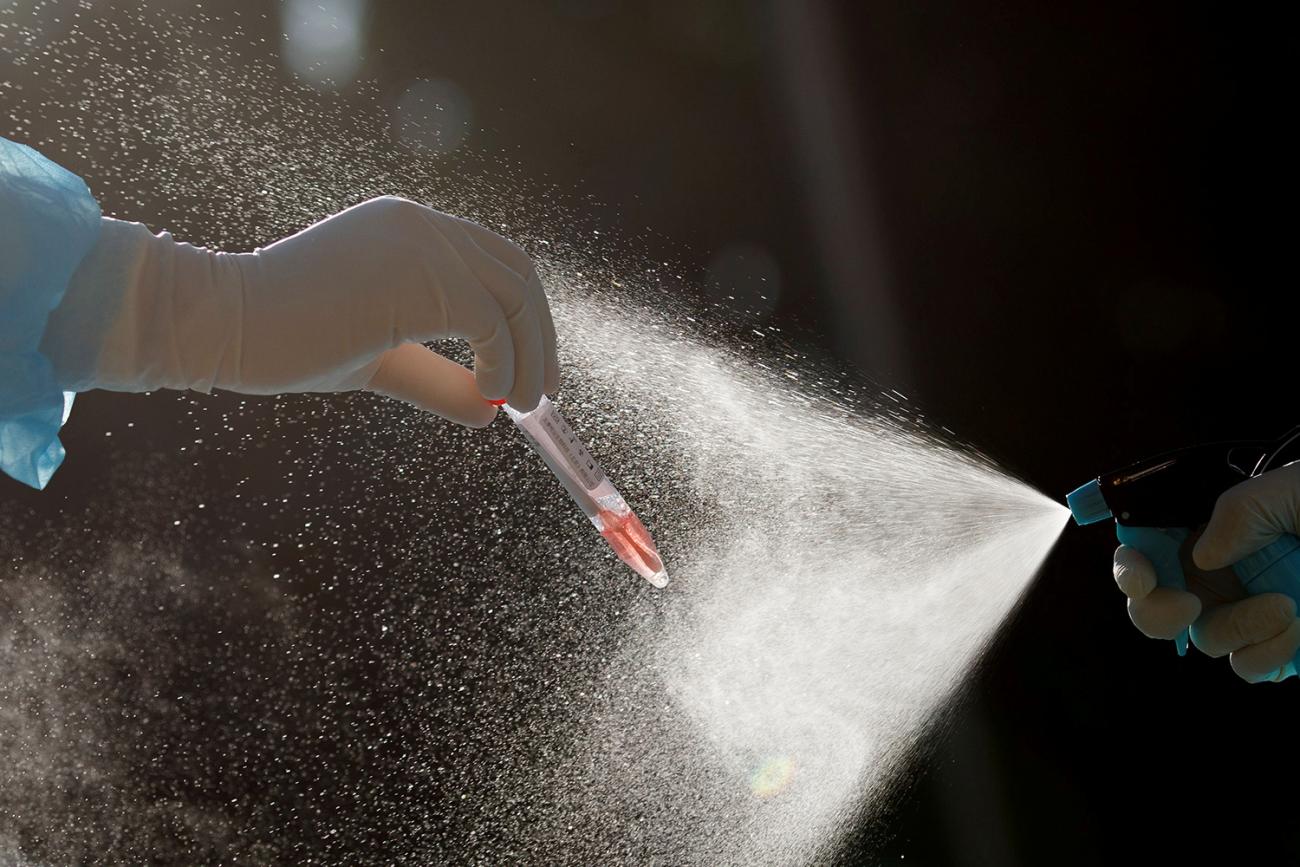
Dealing with Fragmented Data
Unfortunately, many health data systems around the world are grossly fragmented, making it difficult for decision-makers to have a holistic view and act effectively. In addition, critical data that are generated beyond the health care system, including individual and population-level risk factors, are usually not adequately funnelled to inform the observed population health trends. This results in missed opportunities to strategically institute evidence based preventative actions that could save people's lives.
Missed opportunities to strategically institute evidence based preventative actions that could save people's lives
The fragmentation of health data streams takes various forms, including separation along the public and private sector divide, national, regional and subnational governance units, as well as functional splits along health care organizations such health insurance, pharmacies, and diagnostic laboratories—to mention a few. In many parts of the world, health providers within the public and private sectors do not have clear pathways and rules for data sharing and integration between organizations to facilitate speedy analysis and insight generation in response to a public health emergency. To compound the problem, data custodians (even within the same organization) have little incentive to share critical data with their counterparts in other functional areas, even though such sharing could yield better insights for decision-making. Instead, piecemeal analyses using isolated data streams are the order of the day, which means health system stewards often base their decisions on an incomplete picture.
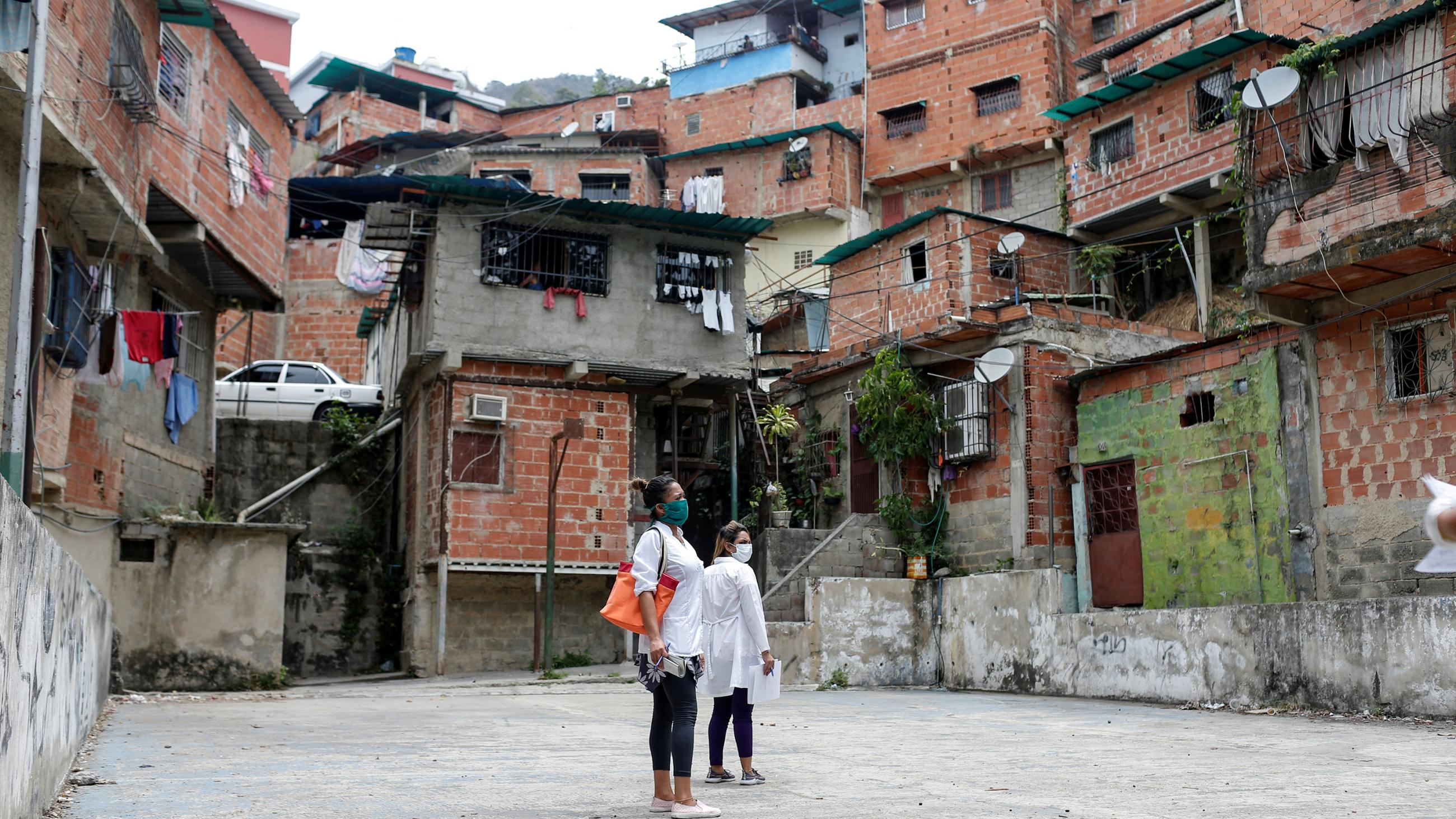
Over time, there have been efforts towards health data integration in different parts of the world. These have been met with mixed results. In most cases, they are often patchy and fraught with lengthy and complex bureaucratic processes to be useful for effective disease surveillance and response.
Isolated and duplicative processes are not only costly but a hindrance
In low- and middle-income countries, data fragmentation is further exacerbated through vertical funding of specific disease programs that often impose specific reporting requirements with little regard for data integration objectives within the broader health system framework. It's not surprising to find a national health system with more than a dozen parallel reporting systems, collecting data for the same disease. Obviously, these isolated and duplicative processes are not only costly but a hindrance towards effective disease surveillance and response.
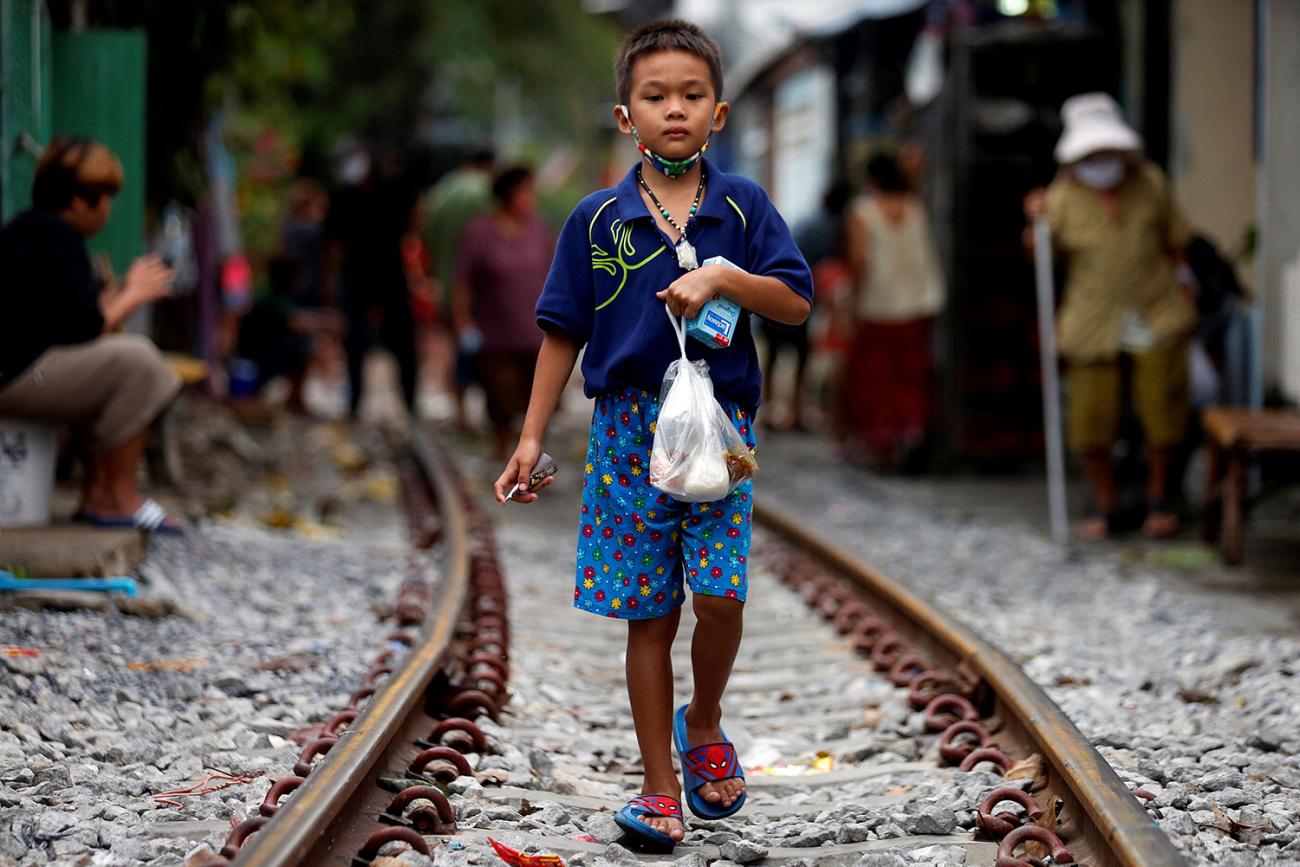
Towards Integrated Health Data
To bridge the health data gaps and improve epidemic detection and response capabilities, countries must move towards integrated health data systems. This would allow for a continuous flow of data from different sources into a joint analytical framework, where different stakeholders could derive useful insights to guide their health actions. The following are critical considerations in the set up.
Stakeholder mapping. Identification and engagement of the key stakeholders who contribute towards the critical steps such as data generation, gathering, analytics, and dissemination is important. The emphasis should be to have complimentary resources and skills, organized into a unique hub or data sharing network, which creates value from such a collaborative environment. For optimal performance, the proposed hub or network should seek to cover data from all the critical components of the health system and beyond.
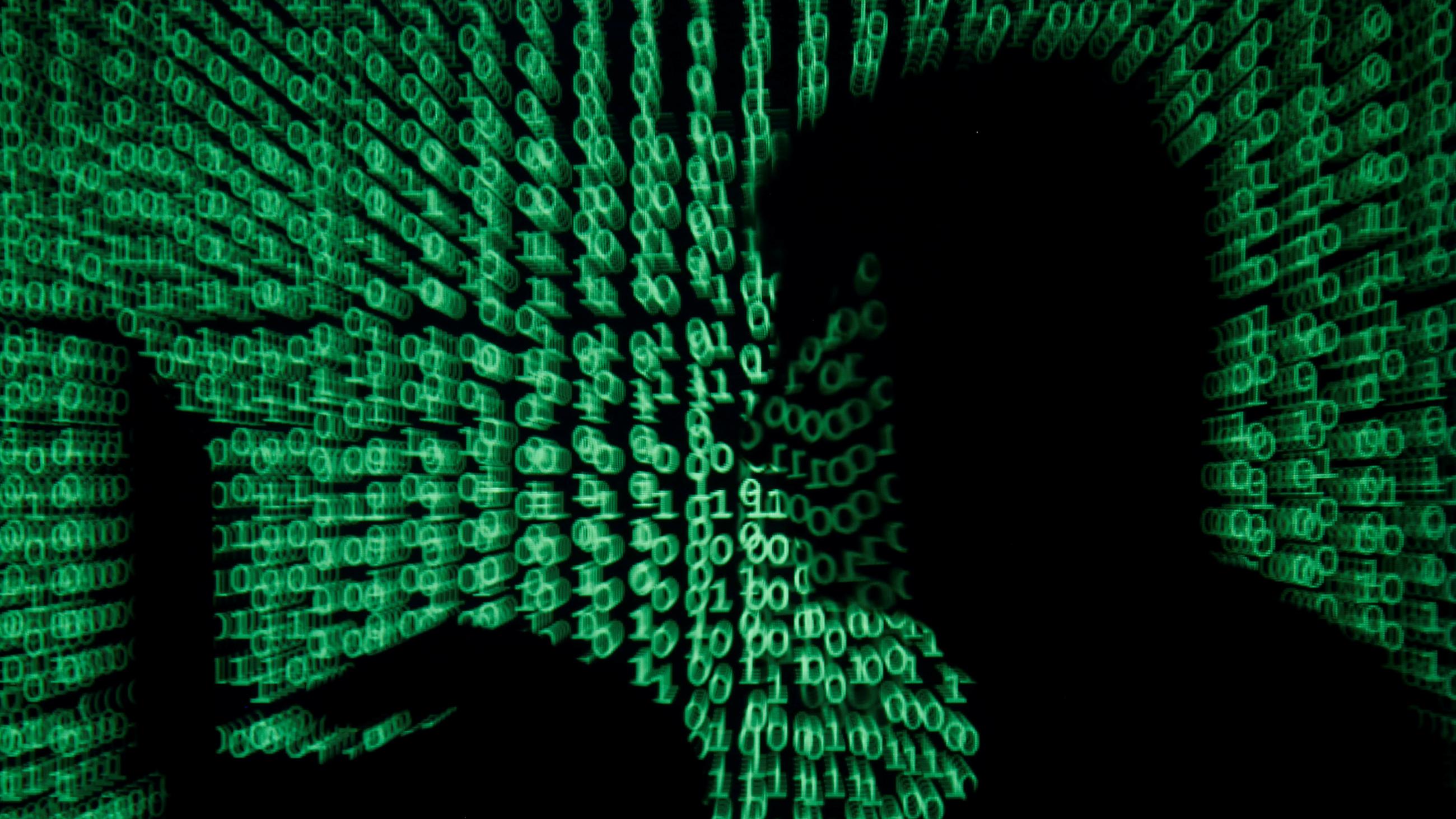
Definition of the regulatory framework. There must be an overarching regulatory framework that clearly outlines the rules of engagement for the different stakeholders to work together effectively. Such regulations should provide a general framework that governs the various steps linked to organizational set up, data gathering, analysis and dissemination, with the aim of developing a robust disease surveillance system that serves the interests of population health. Ultimately, the regulations should be in conformity with the national legislation and international health reporting guidelines.
Definition of the organizational arrangements and incentive structures. For stakeholders to partner effectively through the proposed collaborative hubs or data sharing networks, clarity on roles and responsibilities is paramount. Engagement should be based on complementarity and seek to contribute to the common public health good, while protecting individual rights and respecting privacy. To be fully comprehensive and tap into extended health data sources, it is vital to understand individual and organizational incentives that could encourage and reinforce their contribution. This is particularly true when dealing with individuals and private sector organizations that generate massive amounts of data that could be harnessed for disease surveillance.
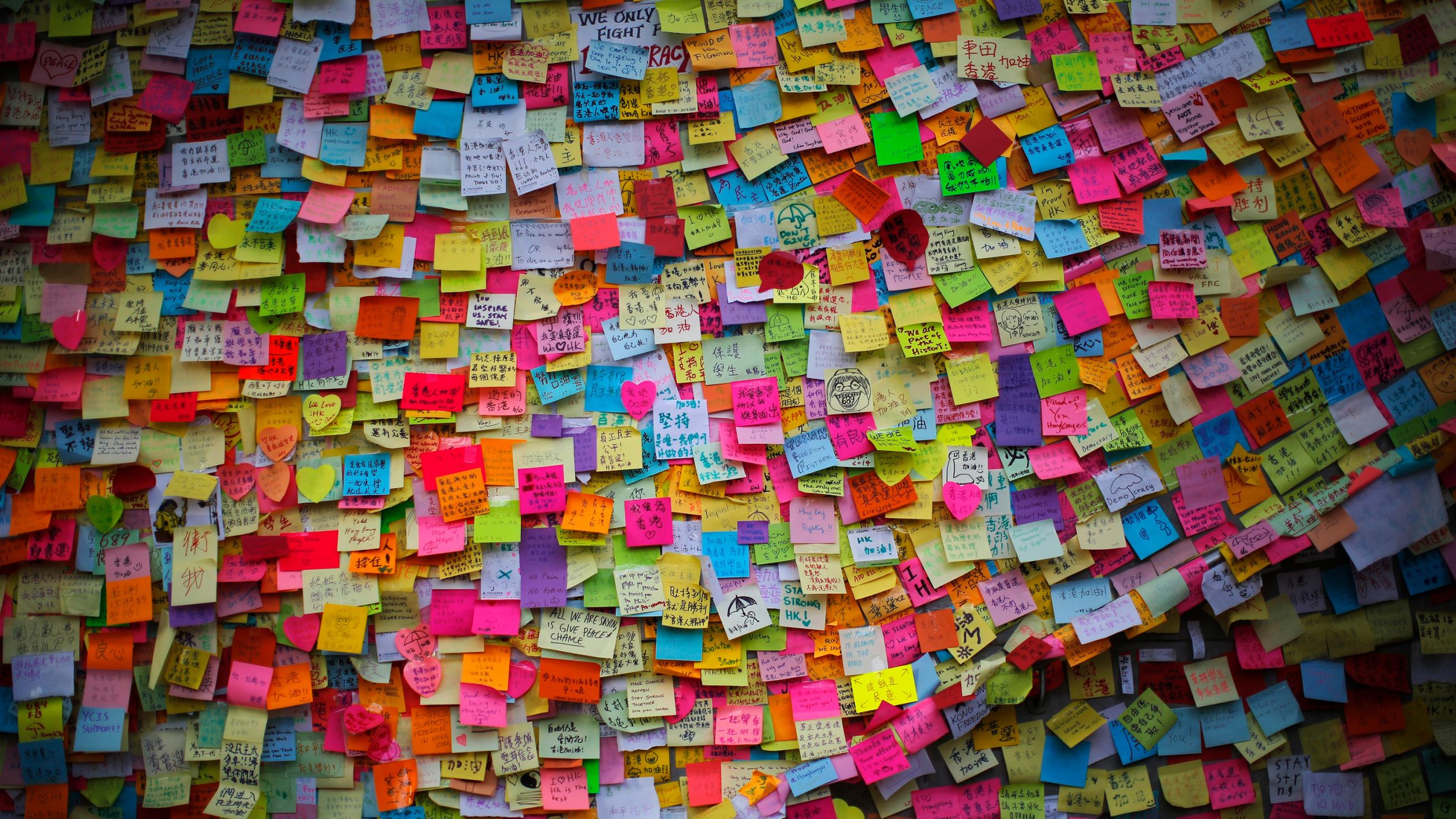
Transparency, mutual accountability and democratic principles of fairness are some of the attributes that could allow for the development of sustainable health data collaborations. For instance, private companies will not be at ease if the data governance structures in place gave unfair advantage to their competitors. Therefore, from the outset, specific objectives and organizational mandates for data sharing should be clearly defined, including issues such as data ownership, governance structures, data access, analysis, and dissemination.
Ensuring stable funding and sustainability measures. The proposed collaborative health data hubs to support disease surveillance are not without cost. In fact, the costs may be quite substantial. Infrastructure, human resources, and technological related costs are significant, particularly for low- and middle-income countries. However, quality data has tremendous value. This can be harnessed in terms of cost savings resulting from improved efficiency in delivery and better health outcomes.
Novel technologies such as blockchain could be leveraged to incentivize various stakeholders, including patients, to share data
With the rapid technological expansion in terms of data generation, storage and capacity to deploy analytical tools, decentralized data access models present boundless opportunities for health system stakeholders to collaborate and advance disease surveillance systems. With the right incentives to involve key stakeholders in the health care value chain, decentralized models could evolve into a sustainable data analytics marketplace, where specific datasets could be seamlessly accessed and analysed by designated parties like companies, clinics or government agencies without incurring extensive infrastructure and setup costs. Further, novel technologies such as blockchain could be leveraged to incentivize various stakeholders, including patients, to share data and capture the value generated from the advanced analytics.
Therefore, as countries reflect on their post COVID-19 strategies, it is imperative for health decision makers to shift their paradigm on disease surveillance systems and evolve in tandem with the changing realities.
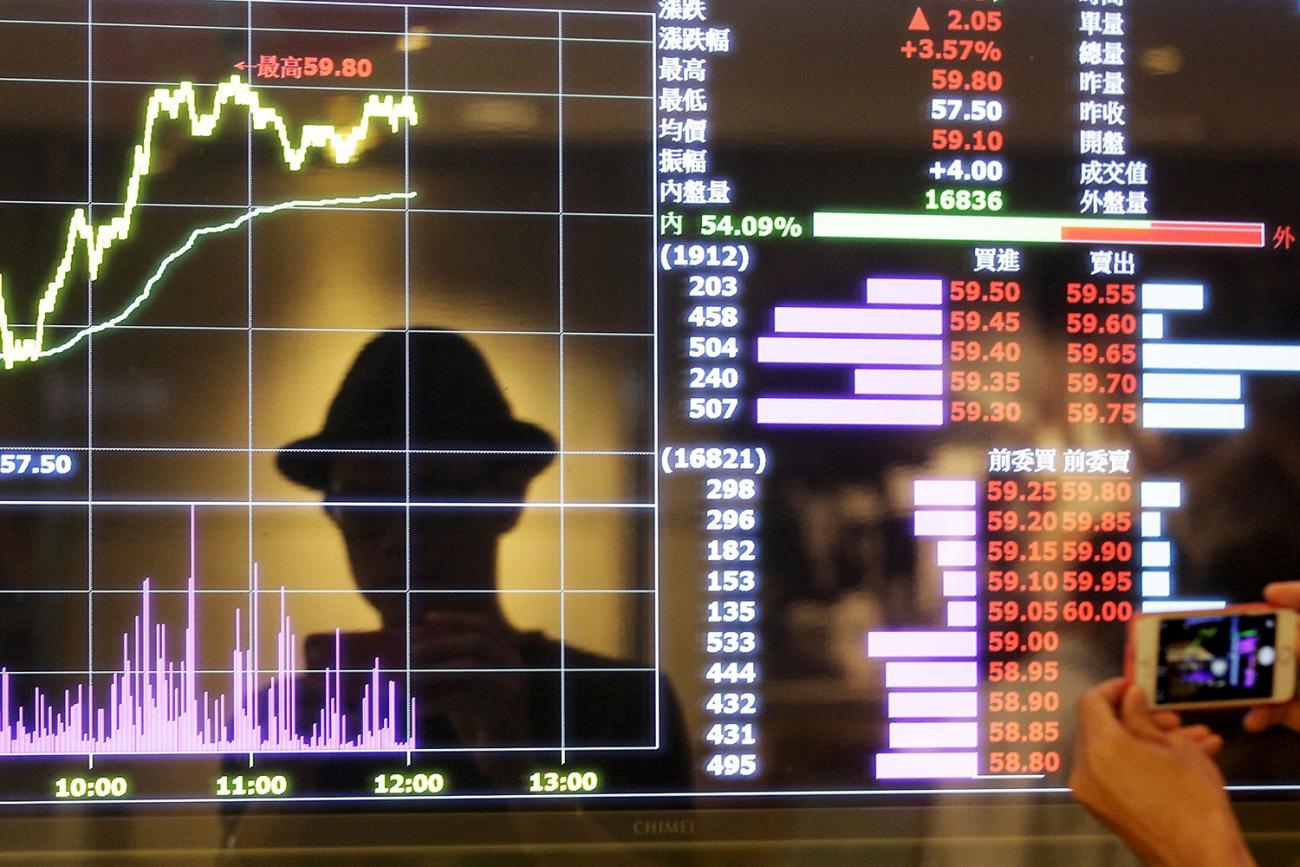
EDITOR'S NOTE: The opinions expressed in this publication are those of the author. They do not purport to reflect the opinions or views of his organizational affiliations or their members.